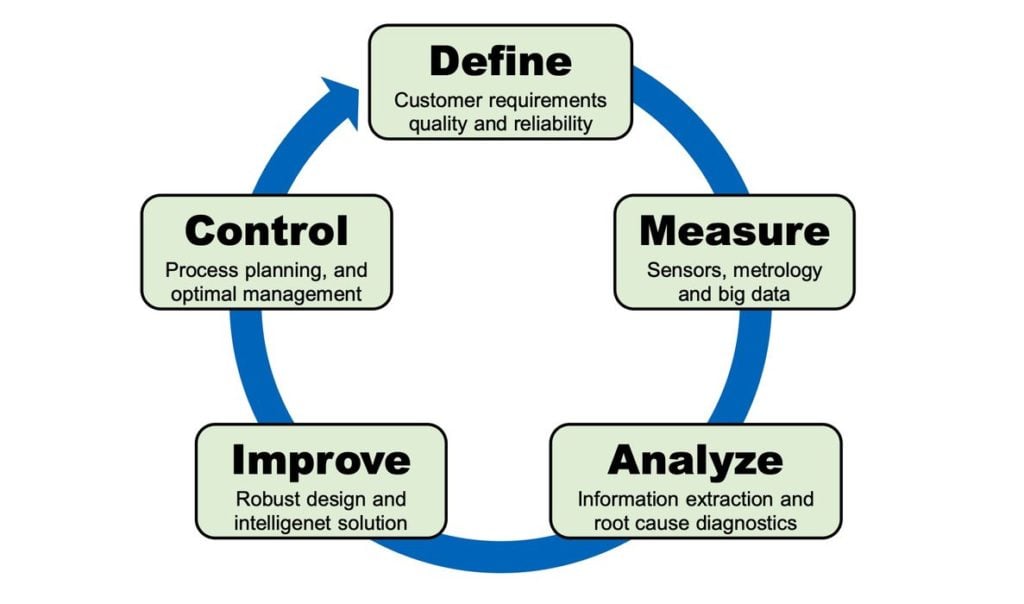
A Six Sigma quality control approach for 3D printing has been developed by researchers at Penn State.
With the increasing use of 3D printing technology for production purposes, the importance of quality control has exponentially risen in the industry. Workshops develop their own QC approaches, while 3D printer manufacturers add sensors and software in attempts to rid production of part defects.
But there could be another approach that wraps up all this work in a consistent way: Six Sigma.
If you’re not familiar with the Six Sigma approach, it is a quality control concept long used in other industries, invented by Motorola in the 1990s. The “Six Sigma” refers to a statistical term where the number of standard deviations from the desired outcome is significantly minimized. In other words, the resulting parts are 99.999% defect-free, or something close to that.
There are two variations of the Six Sigma process. The version used by the researchers, “DMAIC”, involves five steps, described by Wikipedia:
- Define the system, the voice of the customer and their requirements, and the project goals, specifically.
- Measure key aspects of the current process and collect relevant data; calculate the “as-is” process capability
- Analyze the data to investigate and verify cause and effect. Determine what the relationships are, and attempt to ensure that all factors have been considered. Seek out the root cause of the defect under investigation.
- Improve or optimize the current process based upon data analysis using techniques such as design of experiments, poka yoke or mistake proofing, and standard work to create a new, future state process. Set up pilot runs to establish process capability.
- Control the future state process to ensure that any deviations from the target are corrected before they result in defects. Implement control systems such as statistical process control, production boards, visual workplaces, and continuously monitor the process. This process is repeated until the desired quality level is obtained.
The researchers noted that Six Sigma has not really been applied to AM in the past, so they sought to establish a framework through which this could be done.
Why has this not been applied previously? It turns out there is a barrier due to the nature of 3D printing. In traditional manufacturing systems, streams of identical parts are continually produced. The statistical methods used in Six Sigma analyses focus on reducing variability among produced parts, because that’s the goal: identical parts.
But in an additive scenario it’s quite different. Parts can be customized, potentially with each and every instance. That leads to a statistical problem where it’s impossible to focus on reducing variability.
Instead it seems the researchers focused on the process of production rather than the output of production as is done with most Six Sigma analyses. This is perhaps the only way forward due to the problem above.
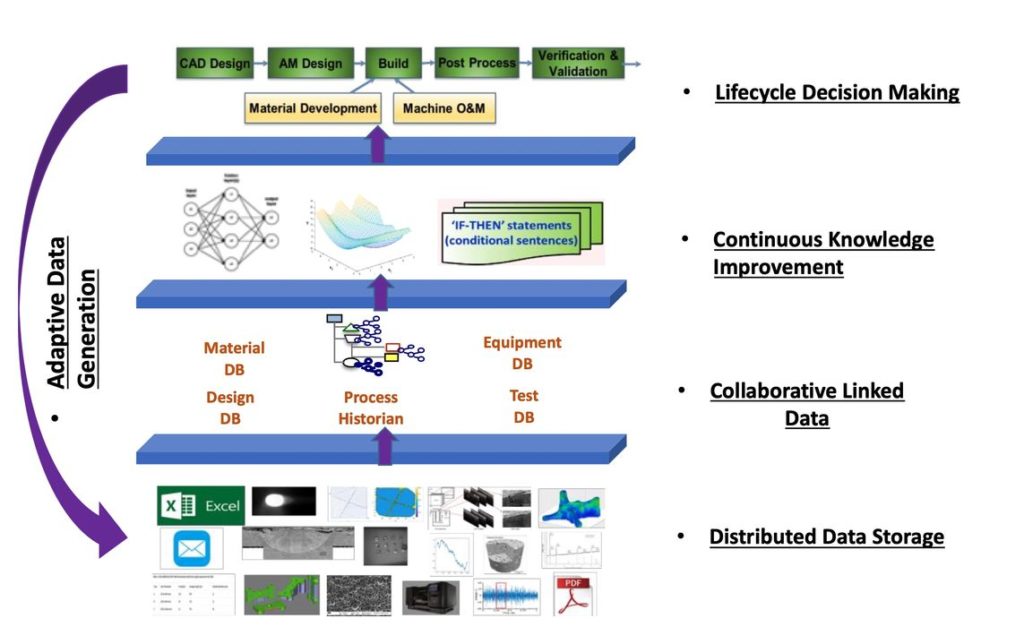
The solution seems to be to leverage the increasingly vast sensor data obtained during the 3D printing process and incorporating it into a standardized data management system. This can then be used for proper statistical analyses and thus lead to detection of defects in a standardize manner.
There’s plenty of details in their paper, and I’m hoping most of the major 3D printer manufacturers will consider looking at this approach. If it were possible to have an agreement between parties on a standard way to approach quality on multiple devices, we could dramatically improve part quality.