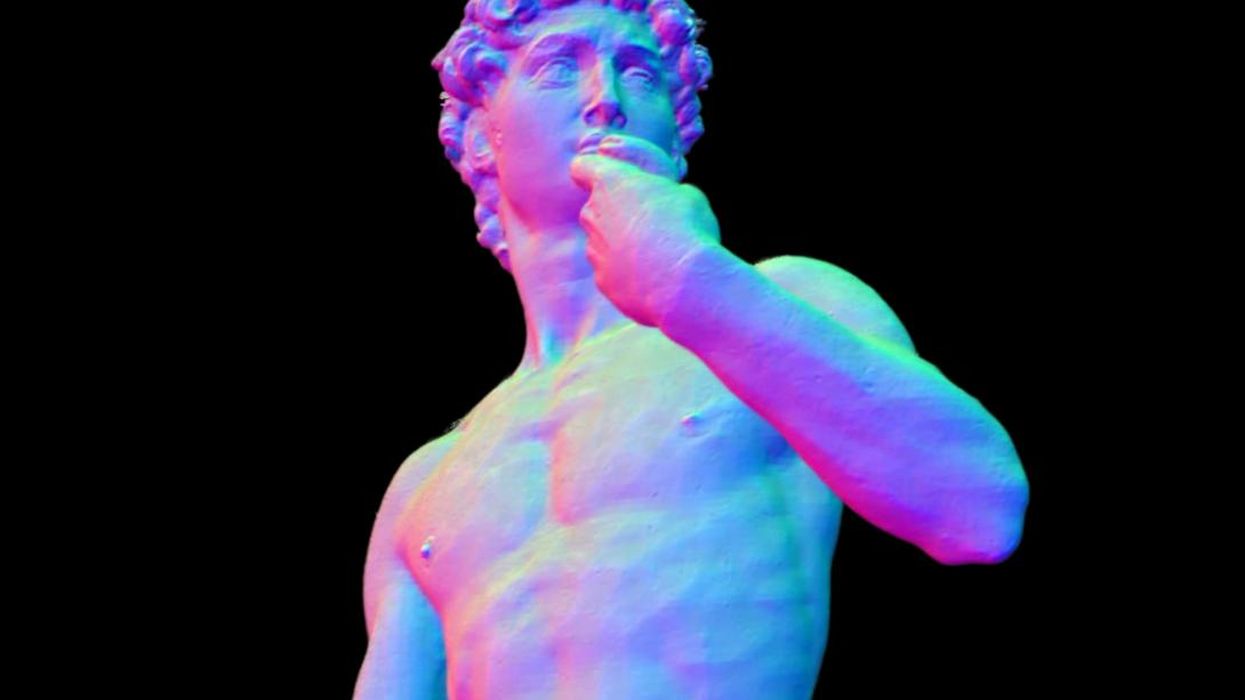
NVIDIA has released new open source code for Neurangelo, a powerful AI-based 3D tool.
This is a surface reconstruction tool, which is software that accepts as input images (or video) of a scene or object, and can then output a 3D asset for further processing. In the case of 3D printing, that would be conversion to a 3D model for printing.
This is a science that’s been around for quite a while now. Photogrammetry is probably the most well-known optical surface reconstruction approach. In photogrammetry a (large) set of still images are taken of a subject from every conceivable direction. These are then processed by special software that detects movement of the background to infer the position in 3D space of scene elements.
Photogrammetry works, but requires a massive number of images — hundreds are typical for good results — and considerable processing power is also required for the software to run. Even then it can take many minutes or even hours to process a complex scene.
There are new methods emerging to perform this activity with different concepts. For example, there’s the NeRF approach, in which only a few images are required and the AI software somehow generates the missing parts of the scene. This works often as good as photogrammetry, but the results are not perfect.
The new software, Neuralangelo, improves on the output quality significantly, as it is able to capture more details and does so efficiently. They explain:
“We introduce Neuralangelo, an approach for photogram- metric neural surface reconstruction. The findings of Neuralangelo are simple yet effective: using numerical gradients for higher-order derivatives and a coarse-to-fine optimization strategy. Neuralangelo unlocks the representation power of multi-resolution hash encoding for neural surface reconstruction modeled as SDF. We show that Neuralangelo effectively recovers dense scene structures of both object-centric captures and large-scale indoor/outdoor scenes with extremely high fidelity, enabling detailed large-scale scene reconstruction from RGB videos. Our method currently samples pixels from images randomly without tracking their statistics and errors. Therefore, we use long training iterations to reduce the stochastics and ensure sufficient sampling of details. It is our future work to explore a more efficient sampling strategy to accelerate the training process.”
How is this accomplished? NVIDIA researchers explain:
“Two key ingredients enable our approach: (1) numerical gradients for computing higher-order derivatives as a smoothing operation and (2) coarse-to-fine optimization on the hash grids controlling different levels of details.”
What does that mean? Honestly, I have no idea. However, it seems to be that they’ve tweaked the algorithms to enable more scale, which transforms into more details.
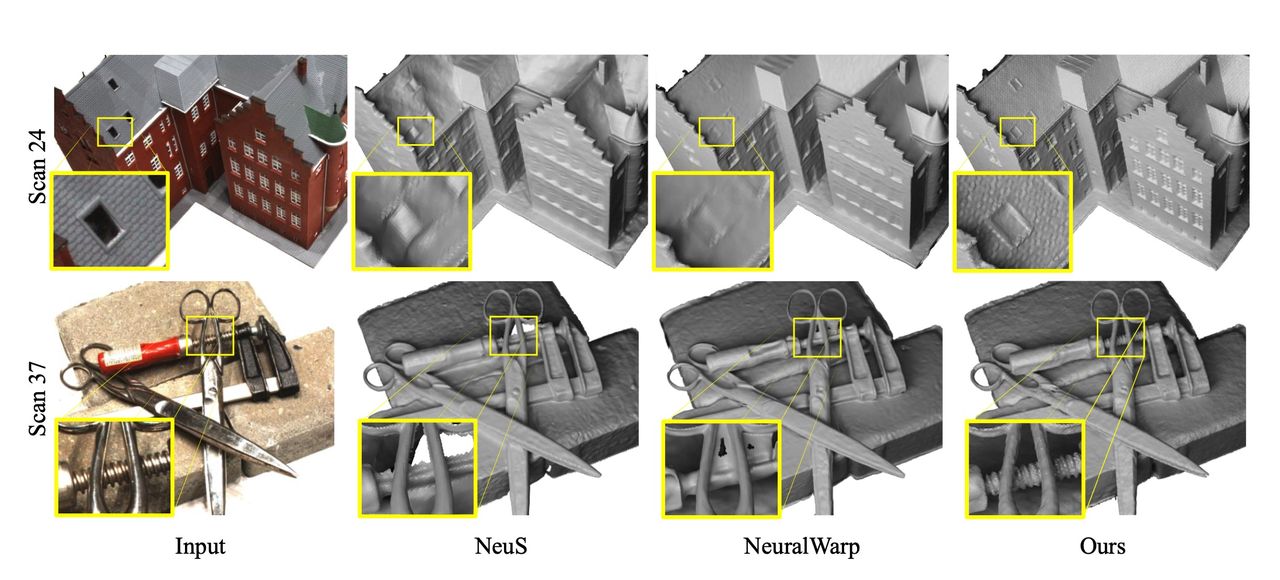
Here’s a comparison of several neural-based surface reconstruction tools, with Neuralangelo on the right. As you can see, the results are notably better than the others. If you look closely, you’ll see that Neuralangelo’s details are incredibly good when compared to the others.
In the NVIDIA research paper there are several examples showing this level of detail can be achieved in multiple scenarios, and it beats the others handily.
How amazing is this? Check out this video from NVIDIA:
What does all this mean? To me it suggests that the neural approach for capturing 3D scans is not in the least exhausted. The improvements made by Neuralangelo are truly impressive, but it seems likely the trajectory for this tech will only get better.
The commercial implications for this are broad. Imagine having a tool in your pocket that can capture massively detailed 3D scans of anything nearby. That could be the world we’re heading towards, one where 3D models are very easily created.
To get there developers will have to dig into Neuralangelo and build new and powerful apps. Fortunately, NVIDIA has now released the Neuralangelo code to GitHub for anyone to use.
Via NVIDIA, NVIDIA (PDF) and GitHub