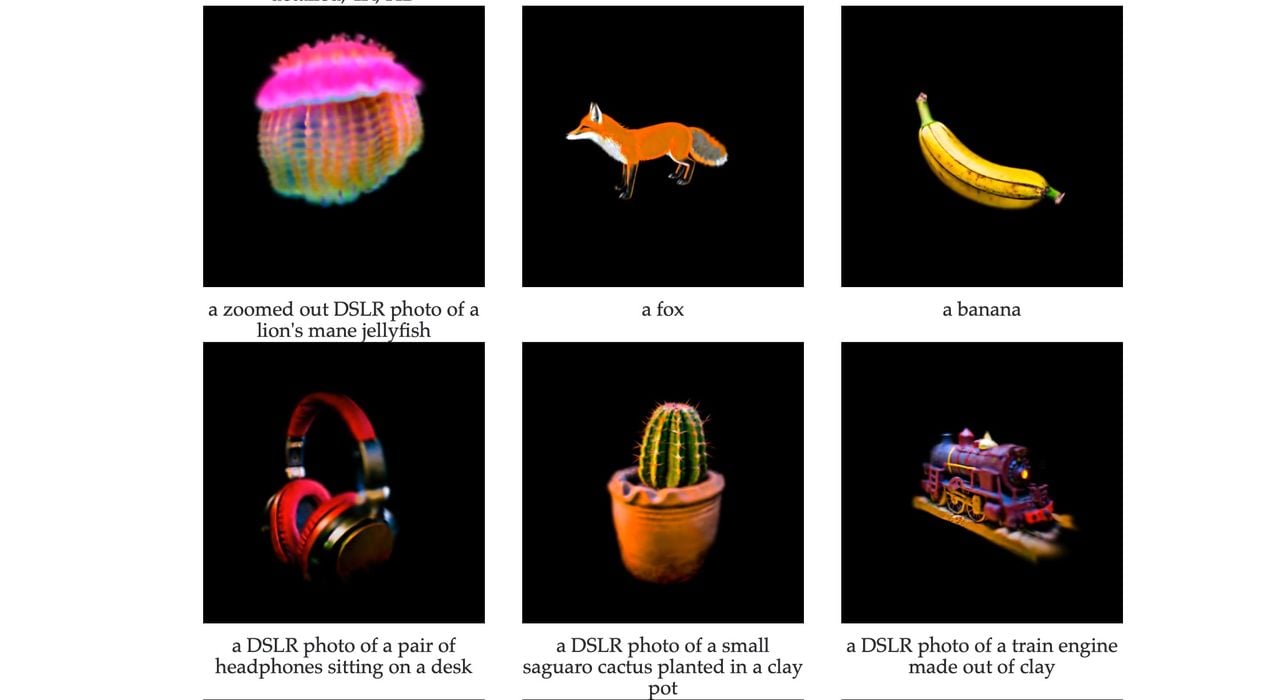
A new experimental text to 3D concept shows a path towards efficient 3D model generation.
“Text to 3D” is one of the new variants of AI-based generative content. Many people are now familiar with the “text to image” concept, in which the user prepares a text “prompt”, which describes the image. The system then uses seemingly magical LLM AI technology to quickly generate a suitable 2D image. Text to image technology has rapidly increased in capability over the past year.
The same base AI technology has been used for a variety of other generative processes. There are, for instance, “text to audio”, “image to image” or “language to language”. Almost anything is possible, and countless AI teams are exploring everything as you read this.
One area of specific interest to our community is the “text to 3D” concept. This is similar to text to image, except that instead of an image, you receive a 3D model.
That’s quite a powerful notion, because the lack of easy access to 3D models is one of the key barriers holding back 3D printing from general use in the consumer space. Consumers by and large are unable to use the complex (for them) CAD tools to create their own designs. They also have trouble using online 3D model repositories because there is either too few or too many models present, and searching is essentially impossible.
If they were able to simply “ask” for a 3D model and get one, there could be huge changes in the future as 3D printer manufacturers would quickly shift focus to the massive consumer market.
That’s why I’ve been watching the development of text to 3D systems over the past few months. It’s actually a bit crazy: this concept would have been completely unimaginable only a few months ago.
The systems I’ve examined up to now have been startling, but honestly not particularly good. The models have been quite rough, and the systems are sometimes restricted to very specific types of models.
Now a new approach might change things. It’s called “GaussianDreamer”.
The researchers developing the software have an interesting approach. They realized that then-current methods didn’t perform particularly well. Their new approach combines two methods. They explain:
“A fast 3D generation framework, named as GaussianDreamer, is proposed, where the 3D diffusion model provides point cloud priors for initialization and the 2D diffusion model enriches the geometry and appearance.”
In other words, they’re using an AI approach on top of another AI approach. This method has been used with great success in other tools, particularly ChatGPT. In that tool skeptics often toss in a query and report a bad result. However, you get better results if you have ChatGPT make new queries for itself and repeat. The same type of approach is used here.
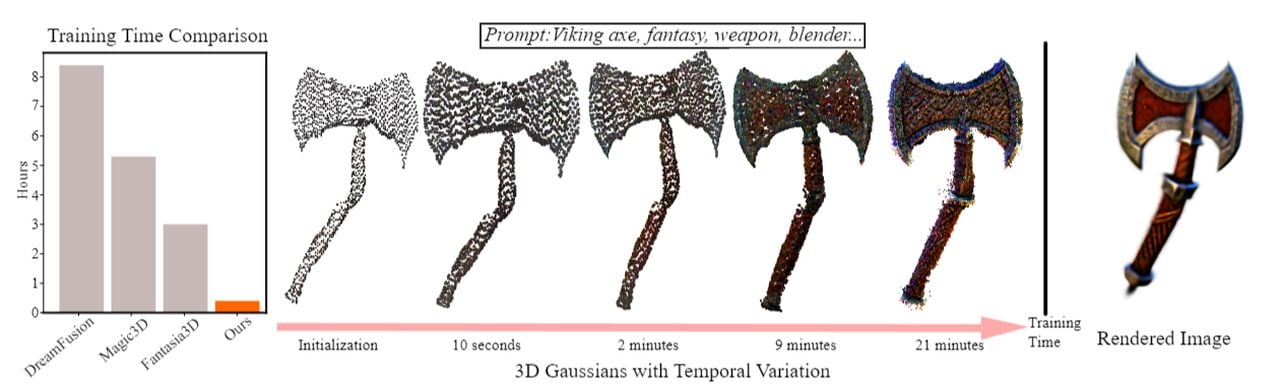
The first stage creates a basic 3D model, and then the new Gaussian Splatter technique is used to refine the generated model. The researchers report that with a single GPU, their software is able to create a “high quality” 3D model in only 25 minutes. If run in parallel, it would be faster. With 32 GPUs the result would be done in less than a minute.
The samples provided on their research paper are indeed impressive. However, all seem to be single objects, which in the 2D image world would be limiting (“make me an image of a walrus eating cereal!”) However, for purposes of 3D printing a single object would actually be desirable.
Again, this is at the research stage and it’s unclear whether this will be commercialized. There is a GitHub repository for the public to give it a try, but you’ll need hardware and programming skills to do so.
Most likely this is just another step on the way toward a very powerful text to 3D concept that will eventually be what we all use.