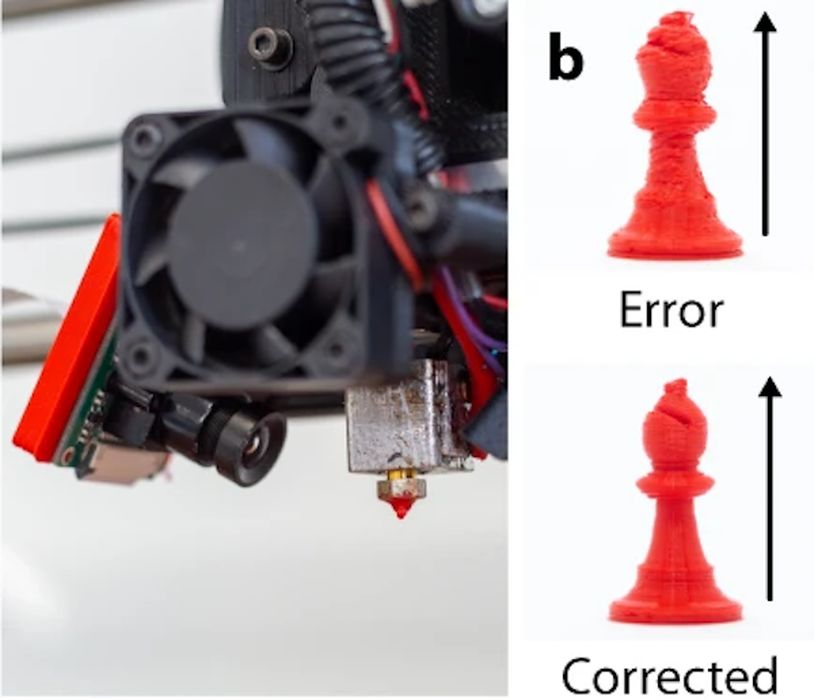
Have you ever hoped your 3D printer would automatically correct errors as it prints? That seems to now be possible.
Monitoring 3D printer progress is not a new thing; itās been done on PBF metal 3D printers for several years, mostly because the cost and risk of failures in that environment are extraordinarily costly. The ability to monitor the meltpool and make adjustments on the fly has saved many failed prints ā and provided quality audit logs for each print as well.
However, none of that type of technology has made its way into the far more common FFF style of 3D printing, which is rife with failures of many kinds.
And those are just the visible errors. What about the hidden flaws inside the printed part that decrease its strength and performance? How can you even detect those without watching every second of extrusion?
Most Fabbaloo readers will have experienced 3D print failures of this type, and at this point have become accustomed to them.
Thatās a bad situation when you have to accept the possibility of failure. The world has many sophisticated solutions, so why canāt 3D printers fix themselves as they print, too?
Now that may be possible.
New research from the University of Cambridge has applied AI to this problem, and it appears they have developed an effective solution.
But how does this work, exactly? The researchers explain:
āWe train a multi-head neural network using images automatically labelled by deviation from optimal printing parameters. The automation of data acquisition and labelling allows the generation of a large and varied extrusion 3D printing dataset, containing 1.2 million images from 192 different parts labelled with printing parameters. The thus trained neural network, alongside a control loop, enables real-time detection and rapid correction of diverse errors that is effective across many different 2D and 3D geometries, materials, printers, toolpaths, and even extrusion methods.ā
This is quite different from the current advanced methods of detection such as Obico/Spaghetti Detective and 3DQueās AutoPrint, which merely detect problems ā they donāt fix problems. These allow the operator to stop the print and reduce waste, but youāre still printing it again.
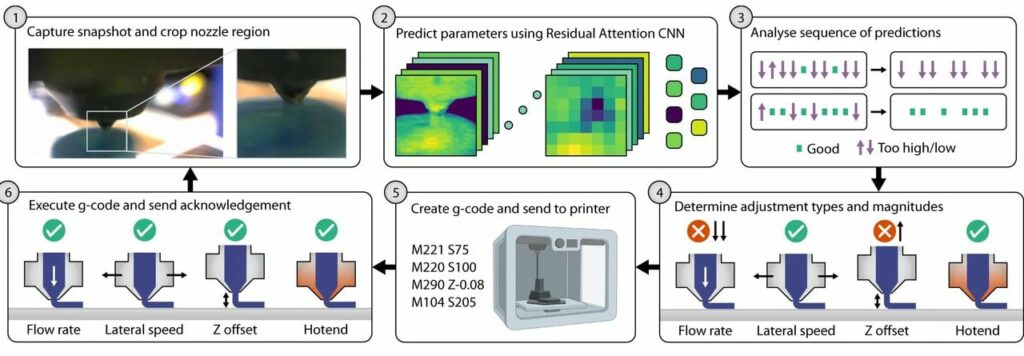
The newly developed approach is to actively correct the problem as the print proceeds. This is very different and should be extremely beneficial. The researchers said:
āFor error detection to reach its full potential in reducing 3D printing waste and improving sustainability, cost, and reliability, it must be coupled with error correction.ā
Their solution involves a set of inexpensive webcams that monitor the print activity from different viewpoints. This image data is fed into a trained neural net that can not only recognize a wide variety of issues, but also knows how to correct for them. Incredibly, they said:
āThe final system is able to detect and correct multiple parameters simultaneously and in real-time.ā
This is unprecedented for a FFF-style 3D printer. While there are several detection systems, none, as far as I know, has an ability to ā in real time ā correct issues.
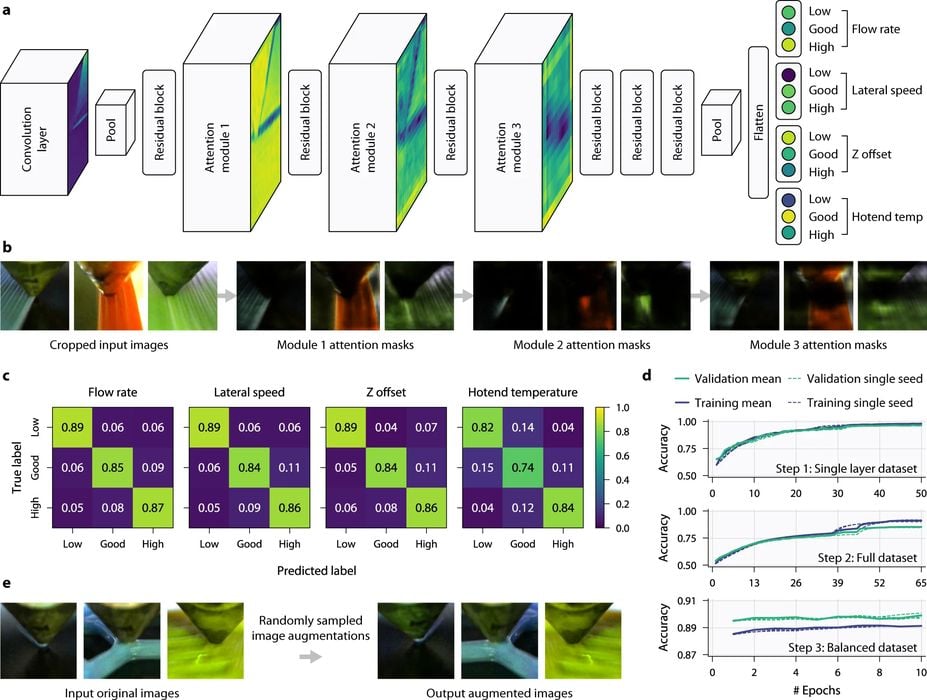
They say the system includes a huge monitoring dataset, and is quite scalable. It is even easily adaptable to many different 3D printers, even ones it has not yet āseenā, as well as being able to learn the properties of different materials.
The system provides a visual āattention layerā that shows operators whatās happening and what the system is adjusting in real time.
The process is fascinating. A special slicer generates toolpath segments of maximum length of 2.5mm. During printing at 0.4 second intervals, the system captures images ā and crucially ā the current print parameters, including temperature, flow rate, position, etc., along with a timestamp. The images are analyzed and the next segment is adjusted accordingly and then sent to the 3D printer. The process repeats until the print completes.
As you might imagine, there are plenty of corner cases to deal with. For example, dark prints cause some challenges with the optical approach. However, it seems the research team has worked through quite a number of unusual scenarios to refine the process.
The researchers believe they can improve the system significantly by, among other things, widening the training set. Since the system is mostly agnostic to sensor types, itās possible to include, for example, infrared sensors that would be able to āsee in the darkā to provide better input data.
This is an extremely impressive project, and shows that it is possible to build an AI into a 3D printer that literally fixes itself every 2.5mm of extrusion. Thatās something every FFF 3D printer manufacturer would want.
To date efforts by 3D printer manufacturers to overcome quality issues has been to refine components, software and materials. But even then, once you push the āPRINTā button, whatever happens will happen, and there is no way to fix the outcome.
A system of this type would solve the problem by having the machine literally tune itself as it prints. Being composed of mostly inexpensive agnostic hardware and some software, it might be possible to build low cost 3D printers that incorporate the functionality.
That could revolutionize the industry. Imagine an inexpensive desktop 3D printer with this technology that could reliably 3D print parts just as good as youād find on much more expensive equipment.
If this research group isnāt considering commercializing this technology, they definitely should. In fact, I would not be surprised if their tech was picked up by one company at high cost just to keep it away from their competitors.
Via Nature