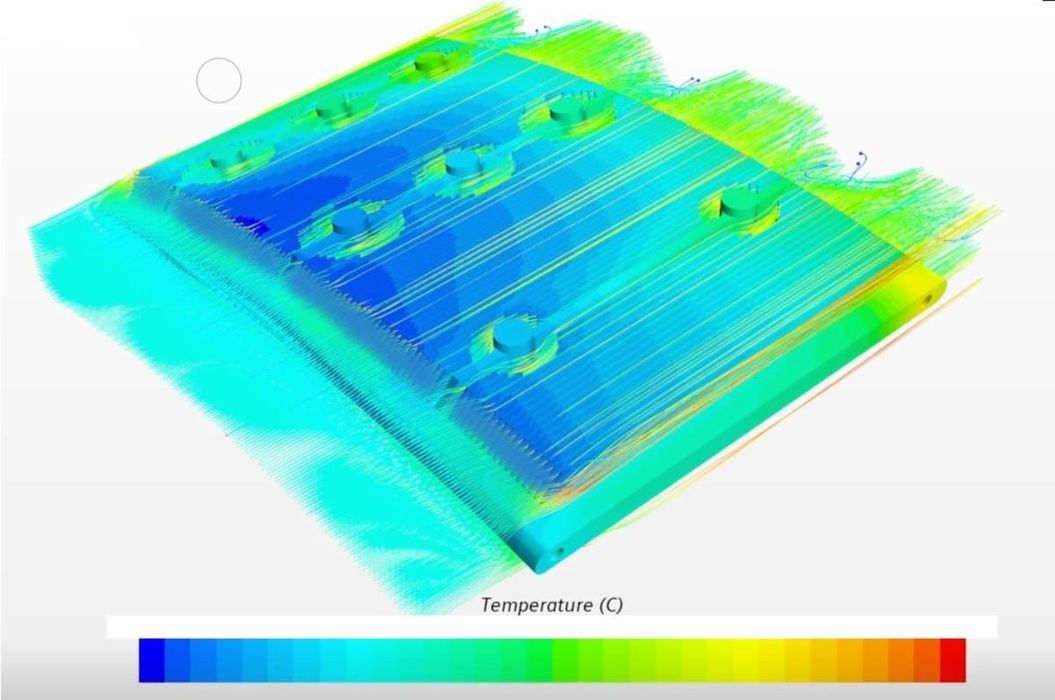
We could see an increase of very unusual yet practical 3D printed parts in the near future due to AI technology.
I’m reading about a startup from last year called “PhysicsX”. The company’s purpose is listed as:
“We are building AI and simulation engineering technologies to reinvent the design and operation of machines and products in advanced industries, with a strong focus on applications impacting the climate and human health.”
It seems they have already been involved in 3D printing with a partnership with Velo3D. The 3D printer manufacturer was having a problem with soot building up on the printer’s observation windows, and was unable to solve the issue with conventional flow simulation tools.
Enter PhysicsX, which figured out a solution, as described here by PhysicsX Founder and co-CEO Robin Tuluie:
“In the Velo3D window nozzle case, a number of metrics were used to automatically quantify the fraction of the recirculating flow within the argon curtain that was travelling upward towards the window. PhysicsX benchmarked the Sapphire window solution at the start of the project, then applied their proprietary AI/machine-learning software, and ran huge volumes of simulations to optimize the final design. This resulted in a nozzle design that produced the optimum Argon curtain flow, while working within the manufacturing envelope of the additive machine.”
That’s quite interesting, but there is more to the story here.
It seems that PhysicsX technology could be used more directly in additive manufacturing to help generate 3D models that are effective and practical to 3D print. Tululie explains:
“AI tools can cut simulation times from hours to only seconds, employing deep learning to automatically evaluate, and then incrementally modify, the geometry of a part—within bounds that the user dictates—in order to create specific outcomes. The resulting, final design achieves the ideal combination of whatever attributes its makers have prioritized: lighter weight, stress and fatigue reduction, optimum fluid flow, heat exchange, conductivity, durability, part consolidation, and more.”
How is this done? Tululie writes:
“AI accomplishes this feat by solving the CFD or FEA equations in a non-traditional way: machine learning examines, and then emulates, the overall physical behavior of a design, not every single math problem that underlies that behavior. This uses far fewer computational resources while achieving an extremely robust evaluation of the design in every applicable environment. Hundreds of thousands of design candidates can be simulated and evaluated in less than a day.”
This technology appears analogous to generating a detailed art piece in seconds vs. having an artist painstakingly draw it over the course of days.
When this technology is commonplace — and I’m sure PhysicsX is hoping it becomes so — we will certainly see many more generative parts developed for 3D printing.
What seems to be different here is that some generative systems will create a 3D model that meets the functional needs as described by the operator, but not necessarily ensure the resulting 3D model is actually printable.
The PhysicsX approach seems to do both, which obviously would be incredibly desirable for those developing advanced parts for 3D printing.
Imagine being able to specify some requirements, and then having a system generate a fully 3D printable model in seconds. This just might become the routine approach in several years.
Via PhysicsX