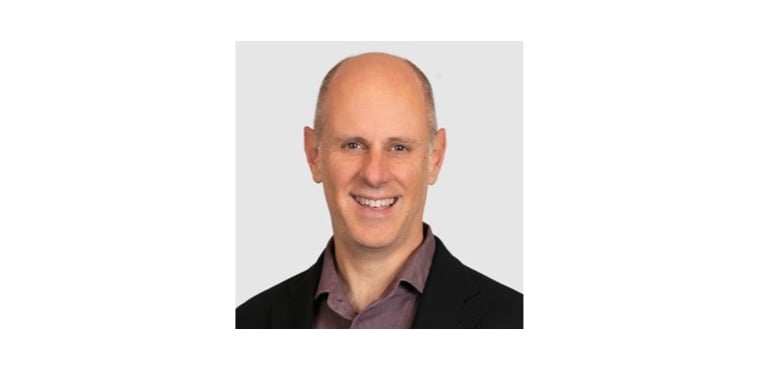
Autodesk implemented AI in 2016 with Design Graph. Nobody noticed.
When we last spoke with Mike Haley in Part 2 of our interview, we were discussing how doing a generative design was, more or less, like doing a finite element analysis. The interface was hardly natural, like what we have come to expect with the natural language interface of ChatGPT and its ilk. We continue the interview from that point.
Engineering.com: Are you saying that AI will finally put the “aid” into computer-aided design?
Mike Haley: Yes. That’s when you’re getting real aid. Let’s look at this in terms of your levels of automation in autonomous vehicles. This is where automation is most germane. For example, automatic dimensioning of drawings. We’re within a hair’s breadth of completely automating that. We’ve launched parts of that. There are things related to that which are low hanging fruit.
It’s reasonably easy for AIs to understand that there’s going to be more complicated but still repetitious actions that our customers in certain industries have. That is going to take a bit longer. It’s their data. The correlations are more complicated. The predictions are of a higher dimension. That means it’s going to take longer.
If 80 percent of the work is repetitive work, maybe over the next 10 or 15 years, there will be a stepwise reduction. Over that time, the repetitive work will have been automated. It’s the same with driving. It will also be stepwise. There is an augmentation side and a creative side, The first augmentation tools are going to be narrow. They’re going to help you finish a sketch of something because they understand what you’re doing.
They will predict the doors or components. Then in another 5 years, maybe it will be able to create the building or the environment it’s in. Maybe it will reference the local building codes. Maybe it will suggest changes needed to conform to those codes with “you might want to redo this because this code thing tends to give trouble in this in this in this municipality.”
Autodesk has a sort of dual personality—one side AEC and the other mechanical. Are you told to focus on one or the other, or are you applying AI to both?
We have a triple personality, actually. We’re also into media and entertainment. And to be honest, it is in M&E that AI is causing the most disruption. Eight years ago, when I was first getting heavily involved in AI, one of the main subjects was how factories were going to be automated.
We were going to have lights-out factories run by robots. But the reverse has happened. The vectors are kind of the same. But the design jobs and the engineering jobs that went into the vacuum transformed the factory.
There are several reasons for that, but one of the primary reasons is that the data is now all digital. Everything is digitized now. Not everything is digitized in the factory. We’re far from that. But from standpoint of a self-respecting design company today, pretty much everything you’re doing is digital. The system might not be perfect, but it’s going to be a digital document. It’s going to be created digitally and cared in ERP [enterprise resource planning] systems. Therefore, the opportunity to train and automate AI is far, far, far greater.
Entertainment has gone digital and so much of entertainment is being generated rather than filmed.
Yes. It’s a total disruption. We are working across all three industries. Our lab has been publishing much of our research. A lot of it has been in the mechanical space because it was easier to get the digital information. We were able to make some big advances quickly because we had data to iterate on.
Speaking of customer data.… I’m reminded of Elon Musk. In his biography, he talks about the wealth of customer-generated video. Tesla has been storing video from the cameras on all of its cars. And instead of creating a situation/response algorithm for every conceivable situation, Musk is going to determine the best drivers and, from their videos, he’s going to train his cars to do what they do. So, instead of having to program every conceivable situation and a response to it, Teslas will behave like the best drivers. That sounds brilliant. Is it possible to do something like that in our world? Can CAD, Fusion 360, in this case, take from the data that is on the cloud and learn from that treasure trove of data it has?
Absolutely. You may remember back in 2016 when we launched a beta feature called Design Graph. It was an AI system that organized your components automatically. It was a shaped-based system. It understood shapes. It was early AI.
Then we did a document system. We mined everybody’s data and organized everything. I gave a talk on it at Autodesk University. We had all the customer data. There wasn’t a single person that was shocked by that. They were saying, “This is fantastic. I hate organizing all my components. If you want to mine my stuff and tell me how my components are organized and help me, you know, with my next catalog, go right ahead.”
I find us in that same situation today. There is a wealth of digital information out there. This is our opportunity to work with our customers to mine all that data and to build the AI. That’s why we’re doing research work in our lab. We’re building the tools and the engines that can take that data and start training on it, learning from it, and predicting it. The trick for us now is how to do this. First off, there’s [the fact] that it’s our customers data. It’s not our data. I don’t want to function like Musk, to be honest.
Read the rest of this story at ENGINEERING.com