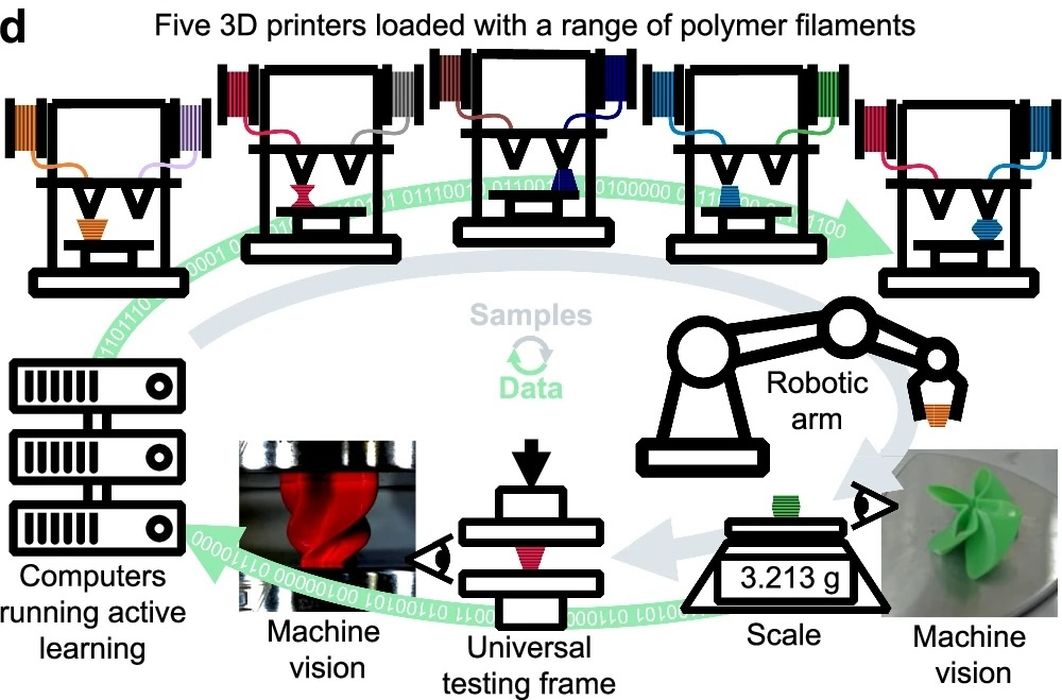
Researchers have developed a “self driving lab”, which can automatically 3D print objects and test them.
The problem under investigation in this research was to determine the most efficiency shape to absorb mechanical energy. One can imagine quite a variety of designs that could be tested, and that could be quite tedious if attempted manually. Here the researchers found a way to perform tests automatically and at scale — without resorting to simulation.
There’s a diagram of the system at top, but here’s how their experiment worked:
- They developed a massive eleven-dimensional parameter space to describe potential 3D models
- Trillions of candidate shapes were generated in the space
- Bayesian optimization methods were used to select 25,000 promising shapes from the candidates
- The 25,000 3D models were queued up for printing on a squad of five standard FFF 3D printers
- When a print is finished, it’s automatically transferred to a test station by a robotic arm
- The same robotic arm then squeezes the print in different ways and measures its energy absorbing capability
- Results are collected and the process repeats for all 25,000 selected 3D models
Whew, that’s a lot of steps, but there’s one thing to notice: it’s entirely automated. In other words, they developed a system that can automatically generate an infinite number of potential solutions and then PHYSICALLY TEST THEM!
Does this seemingly insane setup actually work? It turns out it does! The researchers report:
“The result of this human-SDL campaign is the discovery of a structure with a 75.2% energy absorbing efficiency and a library of experimental data that reveals transferable principles for designing tough structures.”
And it’s not just getting “the answer”; there is a considerable amount of additional data and conclusions possible from running the system. They explain:
“In addition to showing the opportunities for SDLs to overcome design barriers, this campaign results in a vast, labeled dataset that has implications for both mechanics and design more generally. For instance, we explore two high-performing structural motifs and find that they exhibit consistent performance within classes of materials, namely plastic or hyperelastic polymers. Finally, aggregate analysis of this data provides general design heuristics that allow for the efficient selection of materials and structures.”
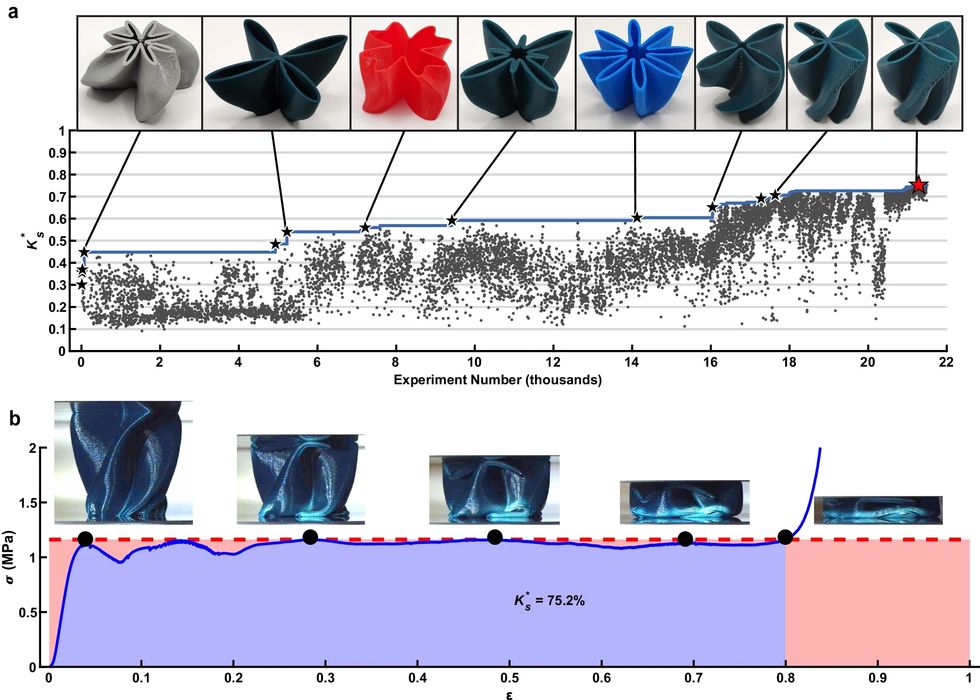
They were able to isolate sections of the data, for example, to determine the best results for particular materials. The best shapes may differ based on the material used. Their test involved seven different 3D printing materials.
Another interesting discovery is that there are relationships between engineering properties of materials:
“Interestingly, all other materials studied exhibit a similarly shaped convex hull with a distinct peak, highlighting both the importance and the feasibility of tuning the material properties to the specific energy-absorbing application. We found that over the seven tested materials, σtp (material peak threshold stress) was strongly correlated with the polymer plateau stress σp, providing an algorithmic process for selecting a material to optimally match use cases across a wide range of threshold stresses determined from different systems to be protected. Then the material can be selected and structured to maximize Ks at that σt. Importantly, we do not believe that σtp is dependent upon 3D printing capabilities or choice of experiments as this value was not found to drift to higher or lower stresses throughout the campaign. As such, this relationship may have applicability beyond the GCS design family.”
The results of this approach might be better than those of a simulation because physical samples were actually tested. This incorporates other factors that might not be included in a simulation, including the variable nature of 3D printing.
Because this unusual approach proved useful, it is almost certainly going to be used for a wide range of discovery projects for many materials in the future.
Via Nature