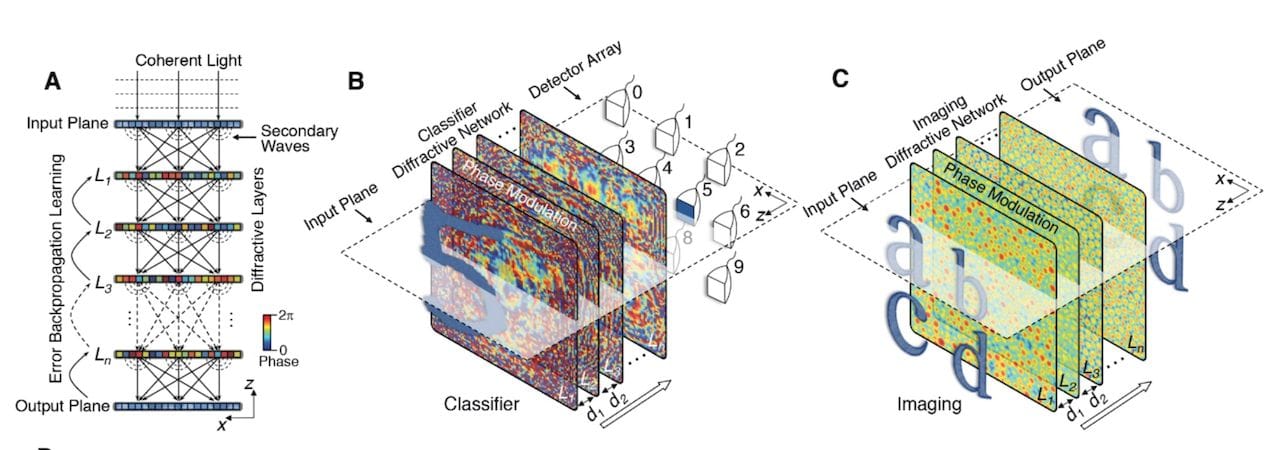
I’m having trouble getting my head around a new concept discovered by researchers.
University of California researchers have developed what they’re calling a “D2NN”, Diffractive Deep Neural Network, a concept that can literally recognize arbitrary light patterns.
Let’s back up a bit, as this is a bit confusing. Neural nets are a digital concept one might find on a computer, and definitely not something in 3D printing, at least until now.
A neural net is a complex algorithm that somewhat mimics what goes on in a brain. An organic brain is a very complex network of zillions of neurons, which you might consider as nodes in this network. As signals (from eyes or other biological sensors) pass through the network, the links between the neurons are strengthened or weakened, making the signal eventually pass through in a consistent path. This is essentially the physical representation of human memory and logic.
By training the brain’s neuron network through repeated exposure to a signal, the brain can learn the pattern.
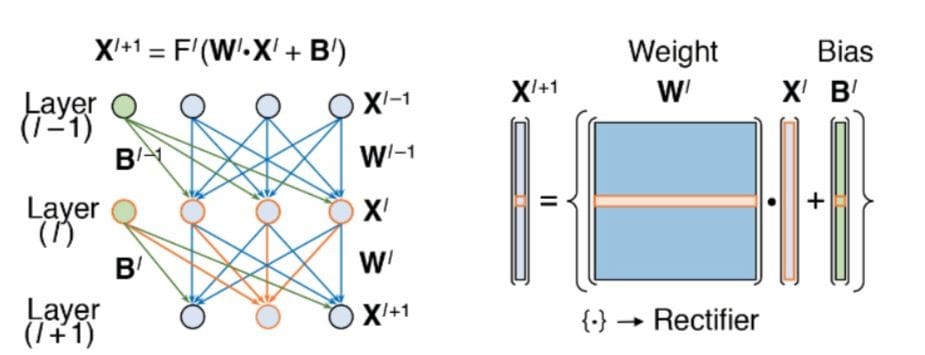
This concept has long been replicated in computer science, where digital inputs pass through a digital neural network – essentially a simulation of layers of neurons – to arrive at an answer.
The way they typically work is that an input signal is provided, and it passes through the network and eventually arrives at a specific output, typically for classification. Imagine a system where you’d show the network an image of a digit, for example, and it would perform all the simulation calculations, eventually lighting up the “9” signal on the other end (assuming a “9” was shown).
These software neural nets have been used for decades in various types of AI systems and have proven quite useful.
But the UC researchers have done something quite astonishing.
They’ve 3D printed physical representations of such networks – and they work!
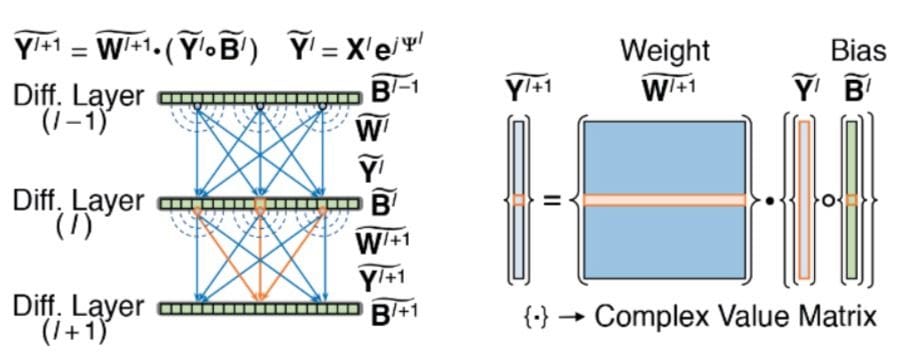
They devised a system of transmissive or reflective elements that approximate the functions of individual neurons in a neural net, and then found a way to 3D print these elements. Then, by replicating 2D arrays of them with 3D printing, they were able to assemble a set of physical layers that pass a light signal in the same manner that the simulated neural net might do.
To make this work they must first develop the neural network in software by training it through repeated exposures. This is a long-understood process, but what is not always understood is the internal structure of the neural net; that develops on its own as the net trains. We don’t know why the internal structure ends up the way it does; we only know the inputs and outputs work. That’s how you have to look at neural nets.
Once they’ve trained a software neural net, they can translate the layers and nodes into physical representations using their transmissive or reflective elements and 3D print it.
The team was able to produce a D2NN device that was able to classify visual images of digits into numbers, for example.
They progressively increased the complexity of the experiments by training more complex and intricate neural nets, 3D printing D2NN equivalents each time. They found that the process does indeed work. One experiment involved a database of fashions:
Next, we tested the classification performance of D2NN framework with a more complicated image dataset, i.e., the Fashion MNIST, which includes ten classes, each representing a fashion product (t-shirts, trousers, pullovers, dresses, coats, sandals, shirts, sneakers, bags, and ankle boots).
This is almost beyond astonishing. Why? Because of what it means.
It means that it is now feasible to produce objects that act intelligently upon exposure to an optical signal. These objects have NO MOVING PARTS, NO POWER REQUIRED and operate at the SPEED OF LIGHT. Once made, they will perform the function FOREVER.
These smart objects could be of incredible value. I cannot start to imagine all the possibilities, but here are a few off the top of my aching brain:
- A sensor pasted on the front of a vehicle that recognizes humans for collision avoidance
- A factory assembly line sensor that instantly determines whether a product is of sufficient quality
- A drone sensor to read street names and house numbers for accurate package delivery
- A courtroom sensor to detect whether a witness is lying or not in real time
And so on.
Anything that can be learned by an image processing system could theoretically be implemented in this way, bringing AI to the level of installable component.
This is indeed an entirely new category of 3D print application.
Via Science