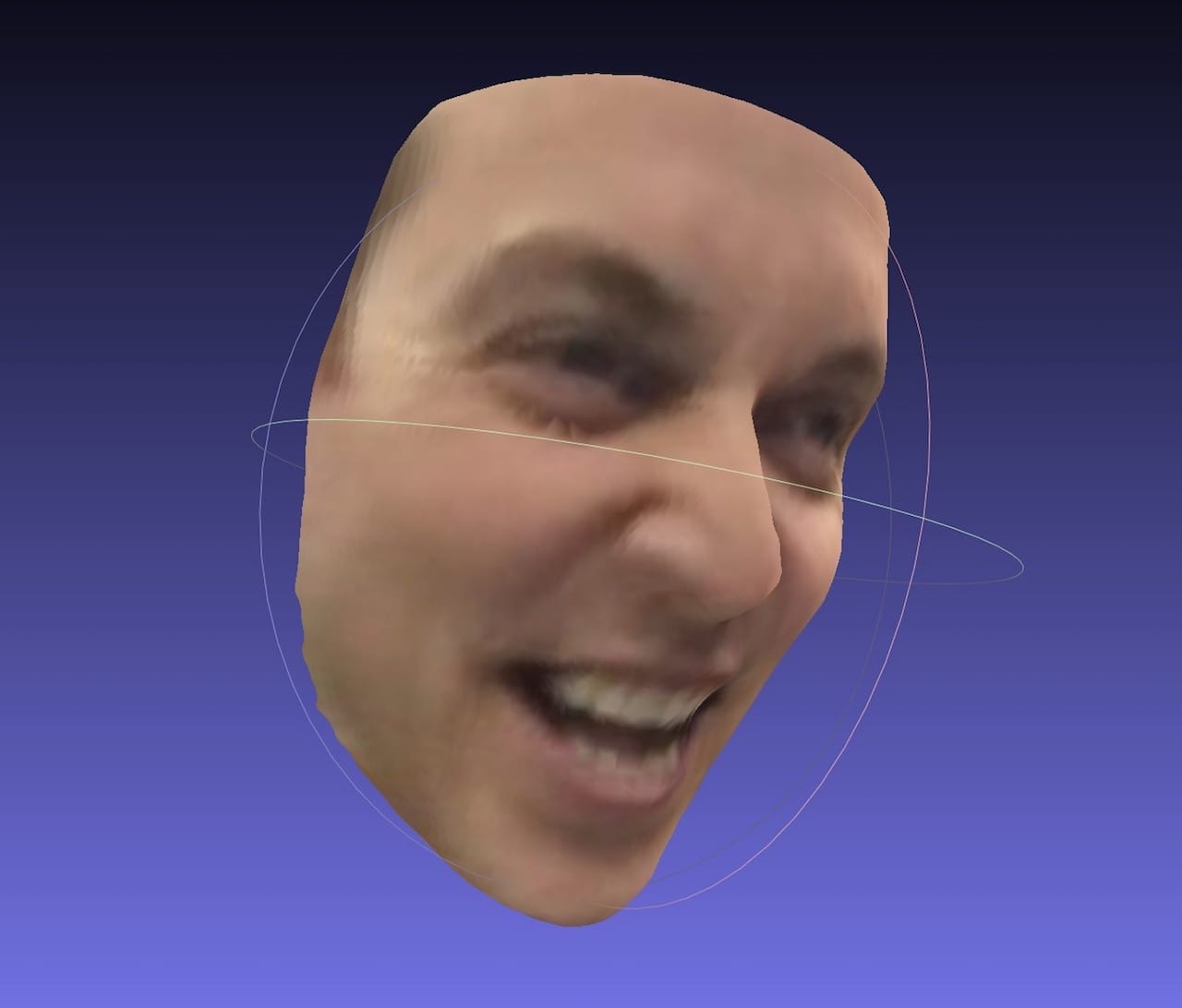
UK researchers have developed a very effective method of producing reasonably accurate 3D models from a single, 2D image.
This is perhaps the holy grail of 3D face scanning: the ability to obtain a 3D model from only a single image. Traditional approaches to producing 3D facial models are far more complex.
Two approaches are conventionally used: a 3D scanner, which projects some form of light on a subject. The light is shone from different directions, with the scanner software interpolating the individual detected point locations to gradually build an accurate 3D model. This approach is usable only by those who have access to a likely expensive 3D scanner and the training to do so.
The other, more common approach, is to use a photogrammetric method in which a series of still images of a subject are analyzed. Images, typically numbering 40 or more, are taken from different positions, up to 360 degrees around the subject. These images are then analyzed by software to see how the image structures shift position relative to the background as different images are examined. This enables the software to identify individual points in 3D space that are then converted into a usable 3D model.
However, that approach requires very careful and comprehensive lighting and a suitable background containing contrasting portions that can be tracked by software.
While possible, both of these methods are well beyond the access by non technical folks.
Now thereās a big change as the UK researchers have created a different approach. Hereās how it works: a single image, usually a front-facing āpassport styleā image is input. This image is run through a convolutional neural network, a software construct that is sometimes used in artificial intelligence and machine learning disciplines. The āCNNā has been trained to match 2D images against a large library of existing 3D facial models.
It seems they are leveraging the fact that faces donāt truly differ significantly in structure; only minor variations really exist. Therefore, this particular implementation would not work if 3D scanning other objects that are not human faces, for example.
However, that doesnāt stop anyone from using the same technique and training the system to produce 3D models of other types of subjects that donāt vary too much in geometry, such as dogs, automobiles, airplanes, etc.
There is a website where you can actually try out this technology by uploading a single facial image. Iāve tested a number of images and found the results to be surprisingly good. From this original image:
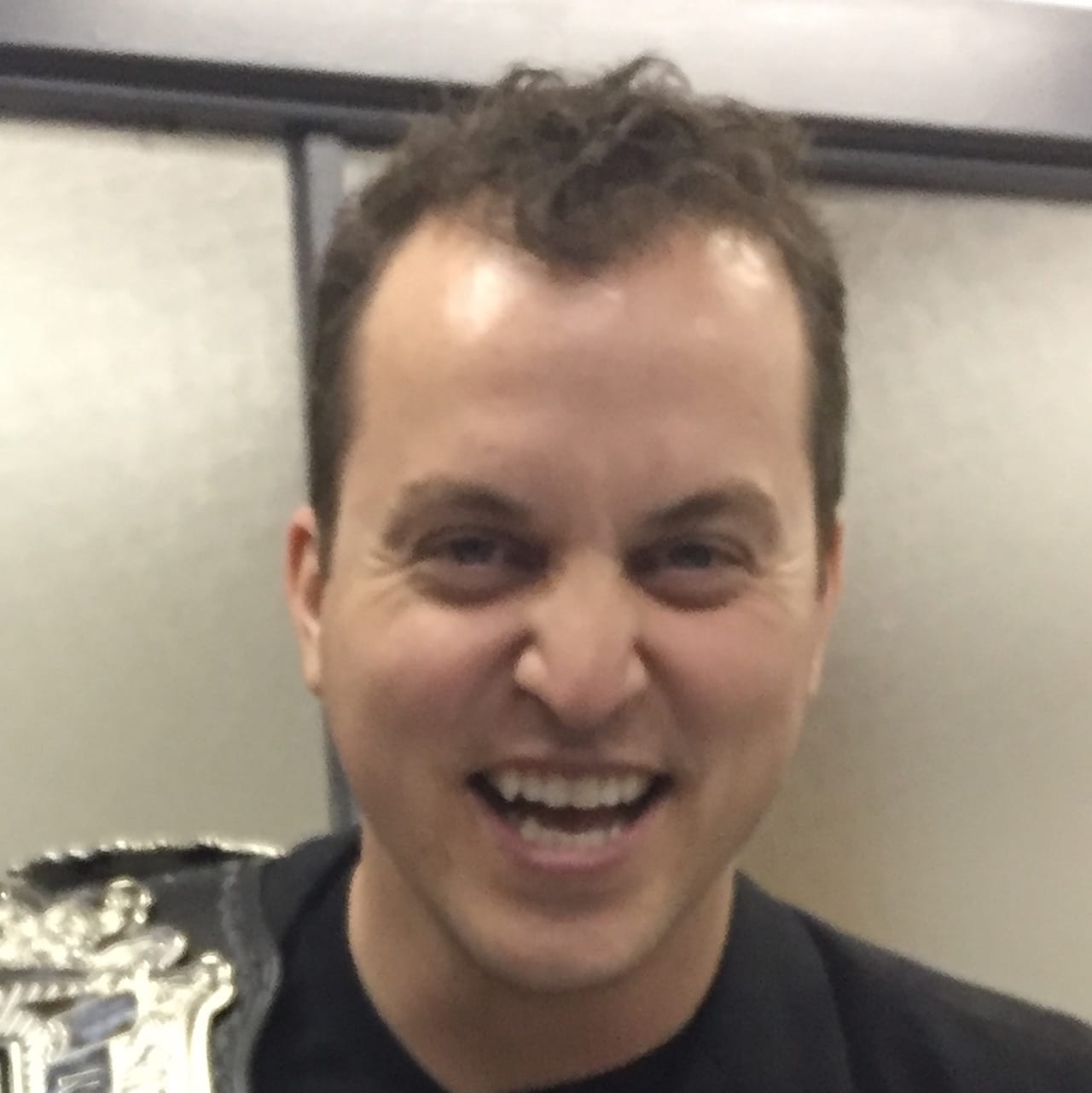
This 3D model was generated within seconds:
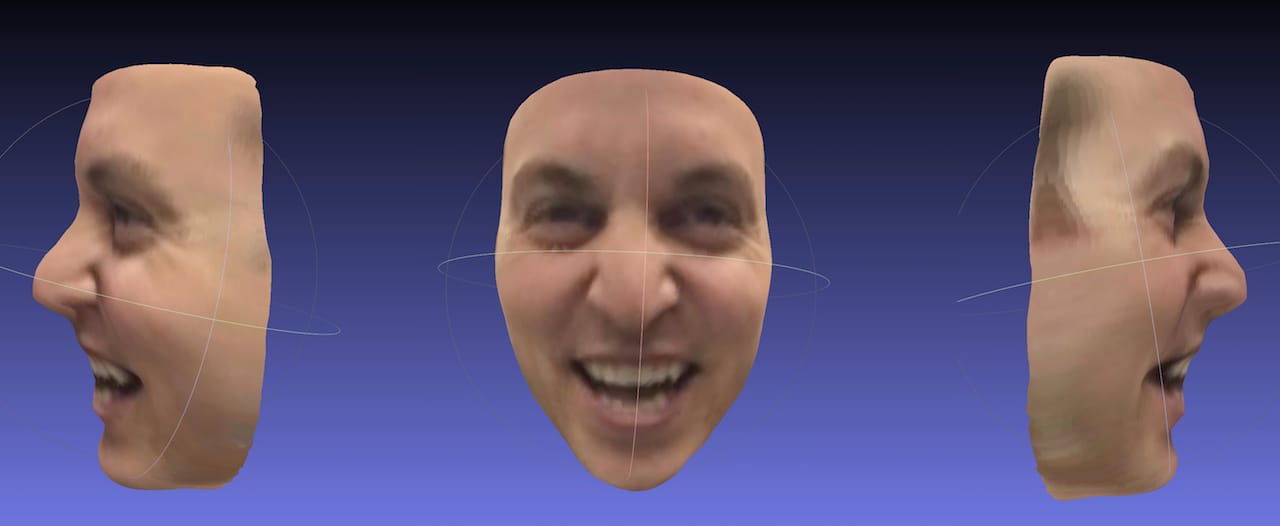
In fact, the resulting 3D models (which are provided in .OBJ format so that color texture information is included). This means you should be able to 3D print these models immediately.
If you happen to have access to a full RGB color 3D printer, you can actually 3D print these models in full color. I think Iāll be trying this soon.
Of course, this is only an interesting research paper at the moment, but I suspect it could easily be turned into a commercial tool that can efficiently recognize a series of image types, beyond mere faces.
Also, the demonstration site may not persist forever and may disappear at any time. However, the researchers have also provided the code on GitHub so you can try this yourself. However, to make it work you will no doubt require a large database of 3D facial models to get it going.
Via University of Nottingham, PDF and GitHub